The rise of artificial intelligence in financial crime prevention
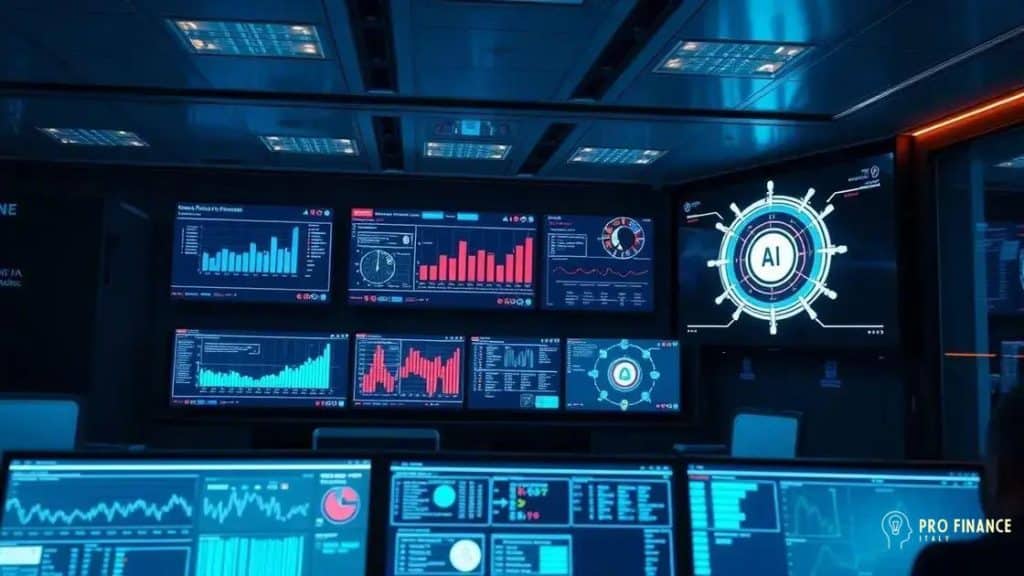
The rise of artificial intelligence in financial crime prevention significantly enhances security by allowing real-time detection of fraud, improving data analysis, and addressing ethical considerations like data privacy and algorithmic bias.
The rise of artificial intelligence in financial crime prevention is reshaping how organizations combat fraud and enhance security. Curious how this technology protects you and your finances? Let’s explore its evolving role.
Understanding financial crime and its impact
Understanding financial crime is essential in today’s world, where technology and finance are intertwined. Financial crime can take many forms, including fraud, money laundering, and cybercrime. Each of these activities poses significant risks not only to businesses but also to consumers.
Types of Financial Crime
Among the various types of financial crime, some of the most prevalent include:
- Fraud: This involves deception to gain money or assets, often through schemes like Ponzi or identity theft.
- Money Laundering: This is the process of making illegally obtained money appear legitimate.
- Cybercrime: With the rise of technology, cybercriminals exploit vulnerabilities in digital systems to commit theft or fraud.
Each type of financial crime has unique characteristics and requires targeted strategies for prevention. Understanding these crimes not only helps in protecting organizations but also supports regulatory compliance.
The Impact of Financial Crime
The impact of financial crime can be staggering. Businesses suffer financial losses, and consumer trust erodes, affecting the market as a whole. Moreover, regulatory bodies impose heavy penalties on companies failing to comply with anti-fraud measures, which can lead to significant financial strain.
Additionally, the fraud landscape is continuously evolving. Criminals adapt to new technologies and exploit any weaknesses in financial systems. This constant change necessitates ongoing vigilance and updated security measures.
Addressing financial crime is crucial for maintaining economic stability. Organizations can implement robust policies and leverage technology like artificial intelligence to enhance their defenses.
Key technologies in AI for crime prevention
Key technologies in artificial intelligence play a critical role in preventing financial crime. With advancements in data analysis and machine learning, organizations can better detect and prevent various fraudulent activities. These technologies empower businesses to safeguard their financial assets more effectively.
Machine Learning Algorithms
Machine learning algorithms are essential tools in financial crime prevention. These algorithms analyze historical data to identify patterns and anomalies. As these systems learn over time, they become more accurate in flagging suspicious activities.
- Predictive Analytics: This helps in forecasting potential risks and fraudulent behavior before they occur.
- Anomaly Detection: AI models can pinpoint unusual transactions that diverge from normal behavior.
- Natural Language Processing: This technology processes unstructured data, such as customer communications and reports, to uncover hidden threats.
Using machine learning not only enhances security measures but also increases operational efficiency, freeing up resources for businesses to focus on growth and customer service.
Facial Recognition and Biometrics
Another key technology is facial recognition and biometrics. These tools offer advanced ways to verify identities and prevent identity theft. By matching unique physical characteristics, businesses can ensure that the person completing a transaction is indeed who they claim to be.
Biometric systems are becoming more prevalent, especially in mobile banking applications. This added layer of security protects customer accounts and establishes trust.
The integration of AI technologies into financial crime prevention strategies is essential for keeping pace with evolving threats. By leveraging these advanced tools, organizations can stay one step ahead of criminals.
How AI detects fraud in real-time
How AI detects fraud in real-time is a pivotal aspect of modern financial security. With the ability to analyze massive amounts of data instantly, AI tools help organizations spot suspicious activities as they occur. This real-time capability allows for immediate actions to mitigate risk.
Real-Time Data Analysis
The first step in detecting fraud is real-time data analysis. AI systems continuously monitor transactions and user behavior. By applying machine learning algorithms, these systems assess patterns and identify anomalies that may indicate fraudulent actions.
- Behavioral Analytics: AI learns what constitutes normal behavior for a user, making it easier to spot deviations.
- Alerts and Notifications: When suspicious behavior is detected, alerts are automatically generated to notify security teams or customers.
- Integration with Existing Systems: AI can work alongside current systems, enhancing their capabilities without requiring a complete overhaul.
This approach not only improves response times but also helps in reducing potential losses from fraud.
Machine Learning and Pattern Recognition
Machine learning plays a crucial role in recognizing patterns in large datasets. By analyzing historical transaction data, AI can develop models that predict the likelihood of fraud. When similar patterns appear in new data, the system can flag these transactions for further investigation.
Moreover, the system continuously learns and adapts to new fraud tactics. This adaptability is vital, as fraudsters often change their strategies to evade detection.
The implementation of AI for real-time fraud detection sets a new standard for financial institutions. The ability to act swiftly makes a significant difference in protecting consumers and businesses from financial losses.
The role of machine learning in financial security
The role of machine learning in financial security is pivotal in today’s digital age. With the increasing complexity of financial transactions, machine learning provides advanced techniques to enhance security measures. By analyzing vast amounts of data, it helps organizations identify threats and mitigate risks effectively.
Automated Threat Detection
One of the most significant benefits of machine learning is its ability to automate threat detection. Traditional methods often rely on predefined rules, which can miss unfamiliar or new types of fraud. Machine learning systems learn from historical patterns and develop algorithms that can recognize anomalies in real-time.
- Pattern Recognition: Algorithms identify complex patterns, making it possible to detect behavior that deviates from the norm.
- Continuous Improvement: Machine learning models improve over time, adapting to new threats and reducing false positives.
- Scalability: As transactions grow, machine learning can handle larger datasets without a decline in performance.
This automation allows security teams to focus on more strategic issues while remaining vigilant against fraud.
Risk Assessment and Management
Machine learning also plays a crucial role in risk assessment. By evaluating customer behavior and transaction history, AI systems can predict potential risks associated with specific users or transactions. This proactive approach enables financial institutions to take preventative actions.
Furthermore, machine learning is valuable for compliance purposes. It helps organizations meet regulatory requirements by providing detailed analyses of financial transactions, which can be crucial during audits.
Investing in machine learning technology enhances financial security frameworks, ensuring businesses can adapt to the evolving landscape of threats. With these advanced tools, organizations are better equipped to safeguard their operations and maintain customer trust.
Challenges and ethical considerations in AI use
Challenges and ethical considerations in AI use are critical topics in the realm of financial crime prevention. While artificial intelligence offers significant benefits, it also raises concerns that need careful attention. Understanding these challenges is essential for organizations looking to implement AI responsibly.
Data Privacy and Security
One of the main challenges is ensuring data privacy and security. AI systems require access to vast amounts of sensitive data. Protecting this information from breaches is vital to maintaining customer trust. Organizations must adhere to strict regulations, such as GDPR, to ensure they are compliant in their data handling practices.
- Consent: Users must provide informed consent for their data to be used.
- Storage: Sensitive information must be stored securely and accessed only by authorized personnel.
- Transparency: Companies should be clear about how they use AI and the data involved.
Failure to prioritize these aspects can lead to damaging consequences for both the organization and its customers.
Algorithmic Bias
Another significant concern is algorithmic bias. AI systems learn from historical data, which can contain biases. If not addressed, these biases may lead to unfair treatment of certain groups. For example, if a fraud detection algorithm is trained on biased data, it may disproportionately flag transactions from specific demographics.
To combat this issue, organizations must regularly audit their algorithms and ensure they are trained on diverse datasets. This effort helps create more equitable systems that treat all users fairly.
Additionally, ethics in AI use extend to accountability. Companies must take responsibility for the decisions made by their AI systems. Clear guidelines should be established regarding who is accountable when an AI system makes a mistake. This transparency fosters trust among customers and stakeholders.
Ultimately, as organizations embrace AI, balancing innovation with ethical considerations is vital for long-term success in financial crime prevention.
FAQ – Frequently Asked Questions about Artificial Intelligence in Financial Crime Prevention
How does AI improve fraud detection in financial services?
AI improves fraud detection by analyzing large datasets in real-time, identifying patterns, and flagging suspicious activities quickly.
What are the ethical considerations when using AI?
Ethical considerations include data privacy, algorithmic bias, and ensuring transparency in how AI systems make decisions.
Can machine learning systems adapt to new types of fraud?
Yes, machine learning systems continuously learn from new data, allowing them to adapt to changing fraud tactics over time.
How can organizations ensure compliance with data protection regulations when using AI?
Organizations can ensure compliance by implementing strict data governance policies, obtaining user consent, and regularly auditing their AI systems.